Внедрение искусственного интеллекта и машинного обучения
AI and Machine Learning Operationalization: Deploying Scalable and Sustainable AI Solutions
Artificial Intelligence (AI) and Machine Learning (ML) have emerged as powerful technologies with the potential to revolutionize industries across the globe. However, to harness the full potential of AI and ML, organizations must focus not only on developing cutting-edge models but also on effectively operationalizing them in real-world systems. This article explores the importance of deploying AI solutions for scalable and sustainable operations, implementing AI models into real-world systems, and the challenges associated with operationalizing AI and machine learning algorithms.
Deploying AI Solutions for Scalable and Sustainable Operations
Deploying AI solutions for scalable and sustainable operations is crucial for organizations looking to derive maximum value from their AI investments. Scalability involves ensuring that AI models can handle increasing workloads and datasets without sacrificing performance. This often requires designing flexible and scalable infrastructure to support the deployment and execution of AI models. Additionally, sustainability focuses on long-term viability, considering factors such as model accuracy over time, data drift, and the ability to adapt to changing business needs.
Implementing AI Models into Real-World Systems
Implementing AI models into real-world systems is a critical step in operationalizing AI and ML. It involves integrating AI models seamlessly into existing business processes, software applications, or infrastructure. This process may require custom development, API integration, or the use of AI platforms that provide pre-built components for model deployment. Successful implementation ensures that AI models can generate insights and make predictions in real-time, providing tangible benefits to the organization.
Challenges in Operationalizing AI and Machine Learning Algorithms
Operationalizing AI and machine learning algorithms comes with its own set of challenges. One significant challenge is the need for high-quality and relevant data. AI models heavily rely on data for training and continuous learning, and ensuring the availability of accurate and representative data can be a complex task. Another challenge is model drift, where the performance of AI models deteriorates over time due to changes in the data distribution or underlying processes. Continuous monitoring and retraining of models are essential to combat this issue.
Another hurdle is the interpretability and explainability of AI models. While complex models like deep neural networks often achieve high accuracy, they can be challenging to interpret and understand. In certain industries, such as healthcare and finance, interpretability is crucial for regulatory compliance and building trust in the technology. Addressing this challenge involves developing techniques and tools for model interpretability, such as feature importance analysis and model explanations.
Lastly, operationalizing AI and ML requires a collaborative effort between data scientists, software engineers, and domain experts. Effective communication and coordination among these stakeholders are crucial for successful deployment and maintenance of AI solutions. Close collaboration ensures that the implemented models align with the business objectives, address domain-specific challenges, and deliver tangible value to the organization.
In conclusion, deploying AI solutions for scalable and sustainable operations, implementing AI models into real-world systems, and addressing the challenges in operationalizing AI and machine learning algorithms are key factors for organizations seeking to leverage the power of AI. By focusing on these aspects, organizations can unlock the full potential of AI and ML, drive innovation, and gain a competitive edge in today's data-driven world.
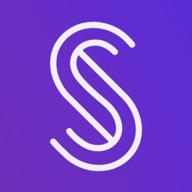
4 Отзыва
Spell is a platform that makes Machine Learning and Deep Learning accessible to teams of any size. An end-to-end data science and machine learning platform, Spell’s infrastructure and tools allow anyone to prepare, train, deploy, and manage machine learning projects. Accelerate Productivity Don’t be held back by infrastructure. With Spell the best CPU…
Узнайте больше об этой компании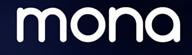
3 Отзыва
With Mona, you gain complete transparency into how your data and models behave in the real world.
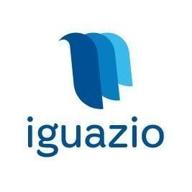
3 Отзыва
It's finally simple to deliver intelligent applications without managing infrastructure.
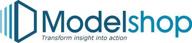
3 Отзыва
Modelshop is a platform that allows organizations to quickly create analytic applications.
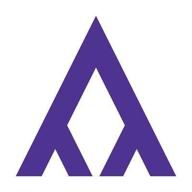
3 Отзыва
Algorithmia is the enterprise machine learning operations (MLOps) platform. It manages all stages of the production ML lifecycle within existing operational processes, so you can put models into production quickly, securely, and cost-effectively. Unlike inefficient and expensive do-it-yourself MLOps management solutions that lock users into specific…
Узнайте больше об этой компании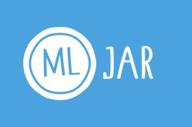
3 Отзыва
MLJAR is a predictive analytics platform. It facilitates machine learning algorithms search and tuning.
3 Отзыва
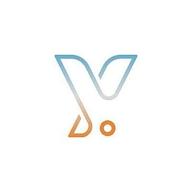
3 Отзыва
At Yields.io, we have created Chiron, our award-winning platform for model risk management (MRM). Our clients use Chiron across all three lines of defence to standardize testing and generate regulatory compliant documentation. This approach increases operational efficiency, leading to lower costs, faster time to market and more collaboration between…
Узнайте больше об этой компании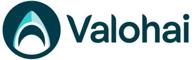
3 Отзыва
Models are temporary; pipelines are forever. Valohai is the only MLOps platform that automates everything from data extraction to model deployment. The Valohai platform makes machine learning in production easy. Data scientists and machine learning engineers can work together to build end-to-end machine learning pipelines that take in new data, train a…
Узнайте больше об этой компании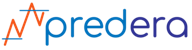
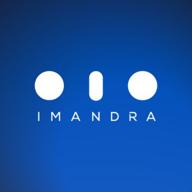
3 Отзыва
Imandra is a cloud-native automated reasoning engine for analysis of algorithms bringing unprecedented rigor and automation to algorithm design and governance.
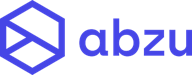
3 Отзыва
Abzu was born from a desire to challenge the fundamental assumptions of contemporary AI, believing that the future of AI lies in the more lifelike simulation of self-organizing systems. If it’s AI, it’s Abzu. Abzu’s pioneering machine learning technology is inspired by quantum mechanics and neural networks, resulting in a transparent and trustworthy AI…
Узнайте больше об этой компании3 Отзыва
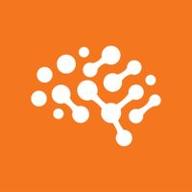
3 Отзыва
Determined AI takes a pragmatic, results-driven approach to deep learning, with a goal of dramatically improving the productivity of deep learning developers. Its integrated AutoML platform simplifies the entire deep learning workflow from data management to model training and deployment.
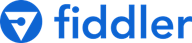
3 Отзыва
Explaining AI outcomes is key to building great AI solutions. When you know why your models are doing something, you have the power to make them better while also sharing this knowledge to empower your entire organization.
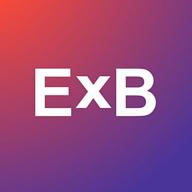
3 Отзыва
ExB offers the only end-to-end ML-driven Intelligent Document Processing (IDP) and Data Extraction solution that automatically processes all incoming digital/non digital documents, invoices, emails (with attachments), customer requests, reports, claims, and any other sort of completely unstructured data to boost automation in input management up to…
Узнайте больше об этой компании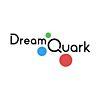
3 Отзыва
We provide bank and insurance sales and customer engagement teams with AI based recommendations that generate new sales opportunities and engage better customers Financial services business teams trust DreamQuark Explainable AI to increase their revenues.
3 Отзыва
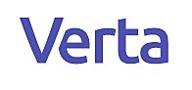
3 Отзыва
AI and Machine Learning Model Management and Operations for Enterprise Data Science Teams
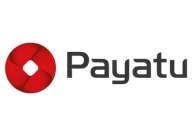
3 Отзыва
Testing AI/ML systems requires domain knowledge. At Payatu, our AI/ML domain experts have orchestrated ways to help you secure your intelligent application against esoteric and potentially severe security and privacy threats. ML Security assessment coverage 1)Understanding the Application a)Use-case b)Product Capabilities c)Implementations 2)Attack…
Узнайте больше об этой компании- Программное обеспечение для операционализации ИИ и машинного обучения относится к специализированным программным инструментам и платформам, которые упрощают развертывание, управление и ввод в действие моделей ИИ и машинного обучения в реальных системах. Эти программные решения помогают организациям оптимизировать процесс внедрения моделей ИИ, интегрировать их в существующие системы и управлять их производительностью и масштабируемостью.
- Использование программного обеспечения для операционализации искусственного интеллекта и машинного обучения дает несколько преимуществ. Это упрощает развертывание моделей ИИ, упрощая их интеграцию в существующие системы и рабочие процессы. Эти программные инструменты часто предоставляют функции для управления версиями моделей, мониторинга и управления, позволяя организациям отслеживать и оптимизировать производительность моделей. Кроме того, программное обеспечение для операционализации искусственного интеллекта и машинного обучения может помочь автоматизировать процессы, улучшить масштабируемость и повысить общую эффективность работы.
- При оценке программного обеспечения для операционализации искусственного интеллекта и машинного обучения необходимо учитывать несколько ключевых особенностей. Ищите решения, обеспечивающие простую интеграцию с популярными языками программирования и фреймворками, а также совместимость с различными облачными платформами. Возможности управления версиями модели, мониторинга и управления производительностью имеют решающее значение для отслеживания и оптимизации производительности модели. Кроме того, такие функции, как автоматическое масштабирование, обнаружение дрейфа данных и объяснимость модели, могут значительно улучшить процесс операционализации.
- Программное обеспечение для операционализации искусственного интеллекта и машинного обучения упрощает процесс развертывания модели, предоставляя оптимизированные рабочие процессы и возможности автоматизации. Эти программные инструменты часто предлагают готовые компоненты и шаблоны для развертывания моделей, что упрощает их интеграцию в производственные системы. Они также могут предоставлять функции для управления версиями моделей, обработки зависимостей и автоматизации конвейеров развертывания, обеспечивая плавное и эффективное развертывание моделей ИИ.